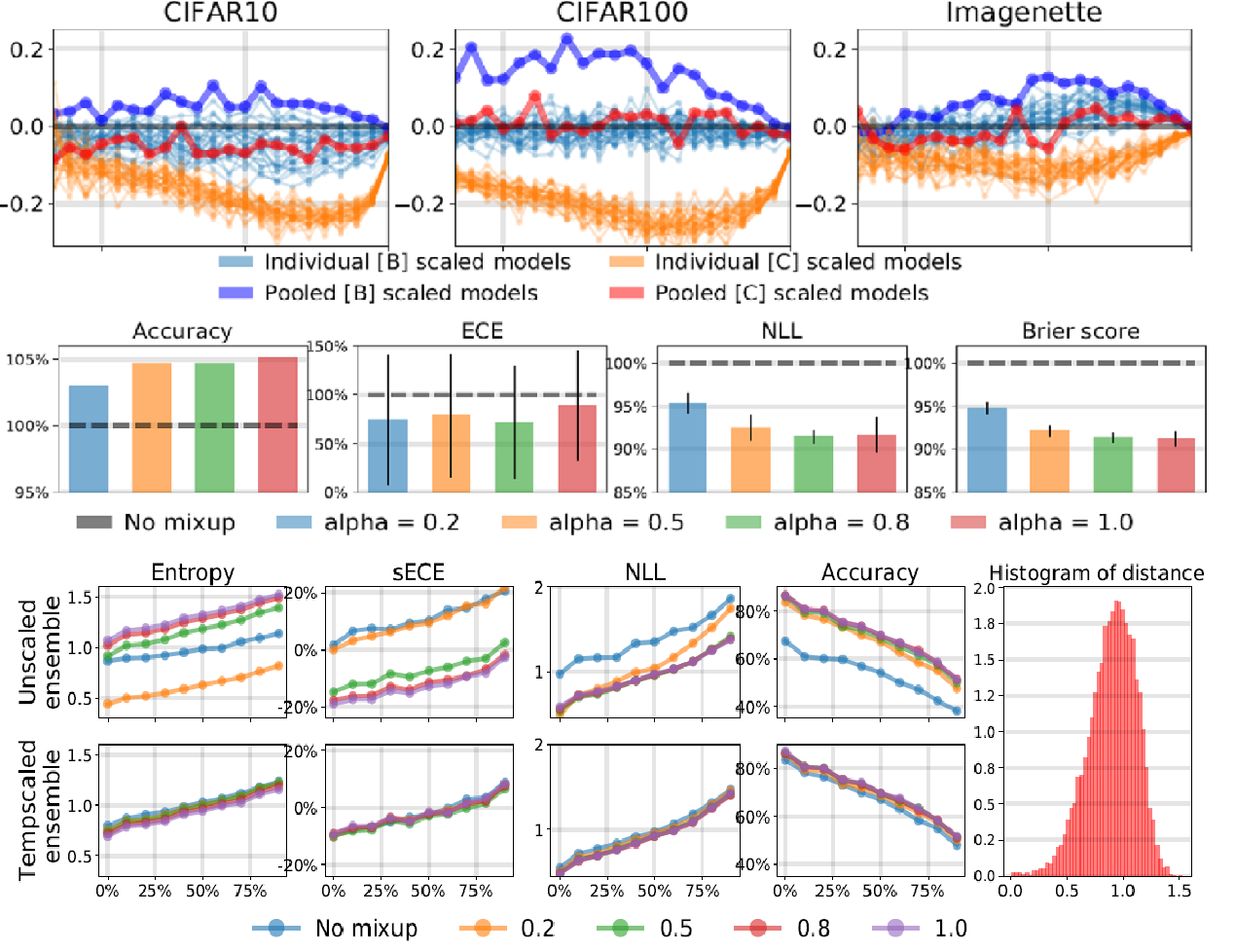
Hi! I'm Rahul.
I am currently a Ph.D. student in the Department of Statistics and Applied Probability at the National University of Singapore. My primary research area is Statistics and AI. Also interested in fun automations, travelling, and sketching.
Research
My research interest spans from Uncertainty Quantification, Unsupervised Learning to Deep Learning based Computer vision with application to Medical imaging, Landmark detection, and Video understanding. Here are some of my completed projects.
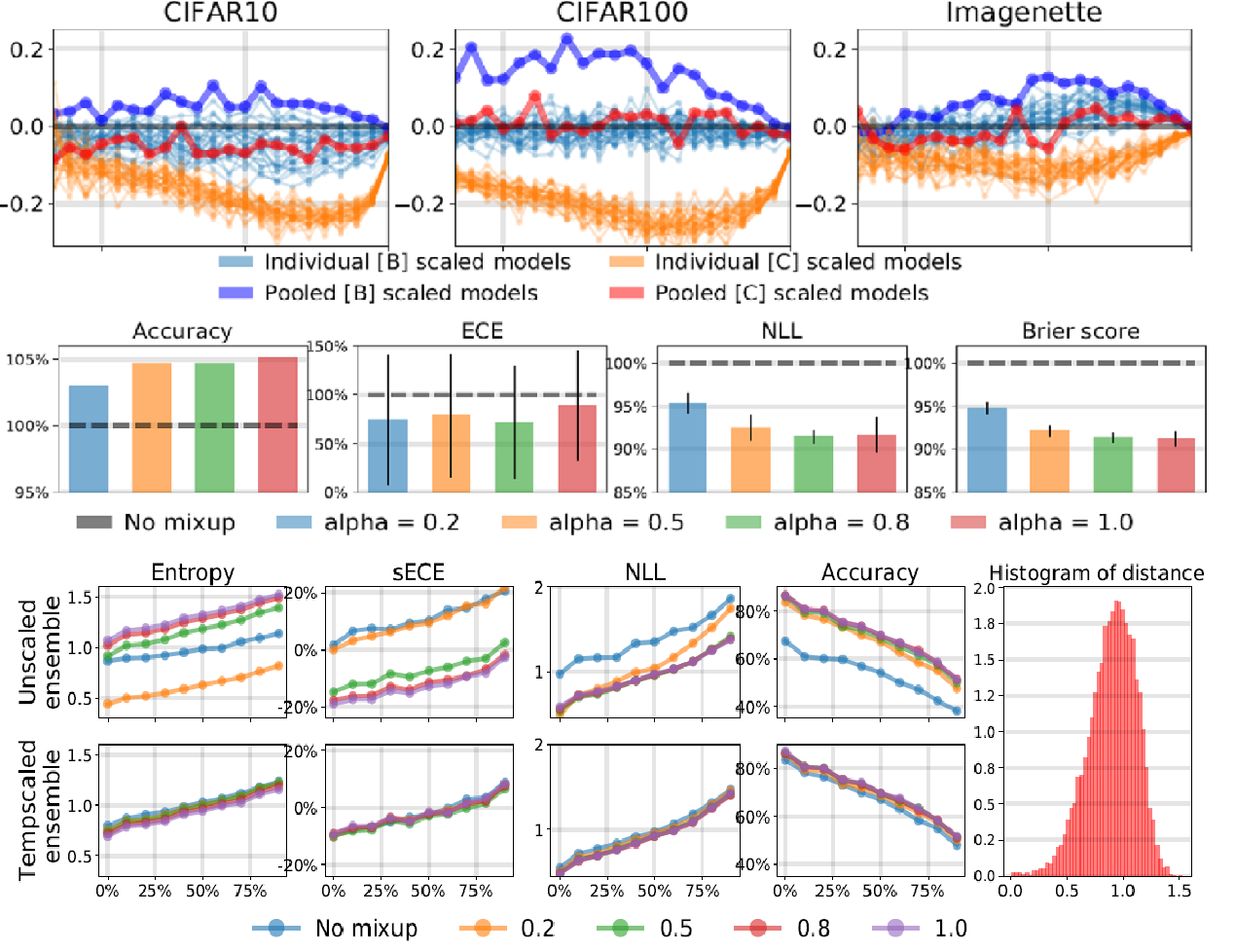
Uncertainty Quantification and Deep Ensembles
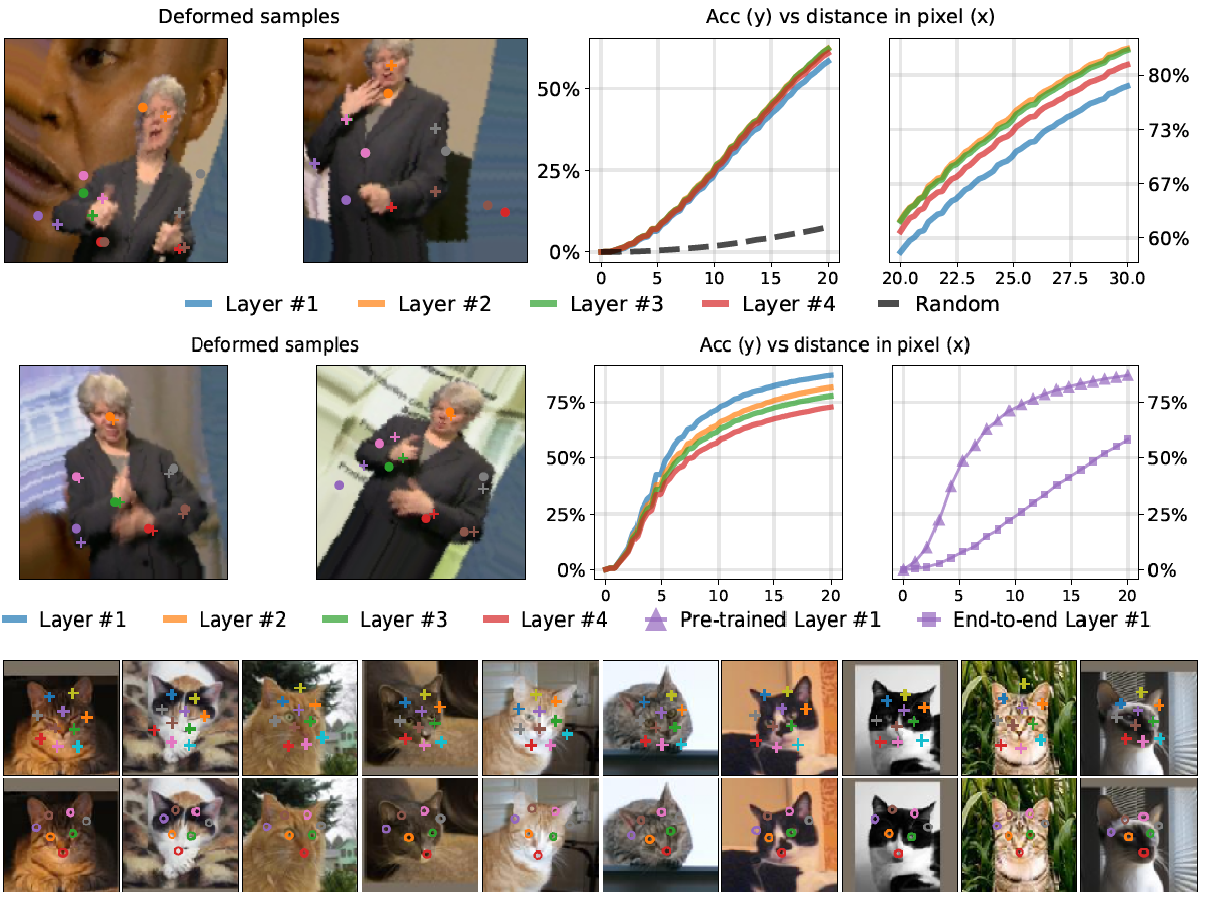
Pretrained equivariant features improve unsupervised
landmark discovery Github
One of the earliest method to discover landmarks in an unsupervised fashion utilized equivariance to image deformations. We investigate one such method and find that the intermediate convolutional features suffer from poor equivaraince. To this end, we propose a two-stage training strategy that utlizes equivariant features trained from contrastive learning.
Ongoing projects
More interesting research works are on its way! Here are some of my ongoing projects.
Video Action Segmentation
It is critical for Action Segmentations in long term videos to be temporally continuous in nature. Current works on video action segmentation relies on multi-layer prediction refinement or auxilliary models to detect action boundaries and combat over-segmentation. We are working on models that do not require such additional tools and yet achieve good Edit,F1 Score as well as Frame Accuracy.
Unsupervised Landmarks and Equivariant features for Downstream Tasks
Many computer vision tasks, especially in the field of Medical Imaging, requires the model to have an overall structural understanding of the full image itself. It is infeasible to endow the models with such high-level understanding by manual supervision. Our unsupervisedly learned image features aim to provide such semantic understanding to any such downstream task.
Presentations
Introduction to Gaussian Processes
This presentation covers the very basics of Gaussian Process, its application and most of the challenges asssociated with Gassian Process models. It was presented by me during my Ph.D. seminar module at NUS.
Oral presentation on Ph.D. progress
In this presentation, I discuss my first project Uncertainty Qualification and Deep Ensembles and also briefly review the literature about Unsupervised Landmark discovery.
Tutoring
I have tutored several modules in the Department of Statistics and Applied Probability at NUS.
DSA1101: Introduction to Data Science DSA3101: Data Science in Practice ST2131/MA2216: Probability ST3236: Stochastic Processes I ST5210: Multivariate Data Analysis
Industry Experience
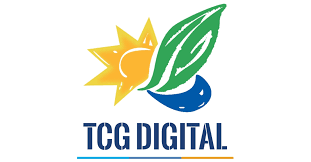
Senior Consultant at TCG Digital
During my 2.5 years at TCG Digital, I worked on a variety of clients and projects from different fields such as Steel manufacturing, Utility distribution to Drug effectiveness testing.
Contact
In case you want to get it touch, my social network profile links are on the sidebar.
Email: rahul.rahaman[at]u.nus.edu
Office: S16-04-16, 6 Science Drive 2 Singapore 117546